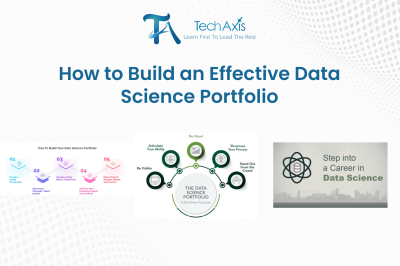
How to Build an Effective Data Science Portfolio
Consider a situation where one has to attend a job interview – the key selling points are made and documented on a piece of paper before the candidate utters a single sentence. That’s the actual strength of an excellent data science portfolio. Looking at the field focused on solving real problems and creating value in the form of new products, a portfolio that reveals technical solutions and showcases how the data is transformed into meaningful outcomes speaks volumes. It is your pass to those all-important employment interviews and ensuring you are not just another resume among many. A data science portfolio is a personal website or a showcase of your projects where people can find out more about one and his/her knowledge in the sphere. On top of it, it gives concrete evidence and experience of the ability to handle data, its visualization, machine learning, and even data storytelling. For a first-time data scientist or entry-level analyst, a range especially multiple projects, for instance, data cleaning, effective modelling or project into a specific field illustrates the flexibility of the scholar or applicant as well as the capability to address real-life issues. Even with the high-tech nature of product development in software engineering, it is advised to include technical explanations, some visuals, and links to code on the GitHub platform in portfolios depending on the specialization level of the portfolio.
Here you will find an explanation of how to design your data science portfolio to appeal to recruiters. We will discover what distinguishes a best data science portfolio, explain how to use it in practice, and share with you templates and examples of great data science portfolios, as well as discuss the basic parts of presenting a portfolio. Everything from choosing significant projects to arranging the layout, you’d learn effective tips for presenting your portfolio that would best depict the potential and make you stand out among other data science candidates in the job market.
Key Components of an Effective Data Science Portfolio
A data science portfolio is not just a mere display of technical expertise, but rather an exhibit of capabilities with actual experiences. Below are the aspects which when integrated make a data science portfolio most effective.
1. Clear and Concise Introduction
As with any introduction, your portfolio’s first page should include a brief on yourself, your skills and what you are looking for.
- Personal Information: Write your name, professional email address, LinkedIn link, and a few words about yourself. This enables any employer to look at the picture and get an idea of who you are from a glance at the picture box.
- Career Objective: Visit the Human Resources Department and identify what specific job position is desired for the future. For example, “Entry-level data analyst who has an interest in using techniques in the profession of artificial intelligence for solving organization puzzles.” This, in my opinion, indicates intent and direction.
- Skills and Expertise: Feature technical skills like programming languages Python, R, SQL, and Machine Learning and domain specializations like healthcare analytics or financial modelling. Such information can be presented in a much more understandable and split way using a bulleted list.
2. Curated Projects
Works or projects are the major parts of a data science portfolio as they prove one’s worth or competency.
- Project Selection: Select 3–5 significant projects that would make a difference in your working profession. For instance, an ML engineer might use projects that involve the use of predictive models or deep learning.
- Project Description: For each project, state and explain the goal briefly in 1-2 sentences. The problem statement which you have solved is. What approach you have used in the specific steps as data cleaning, feature extraction, or model choice? The outcomes and the knowledge you gained. When you describe a benefit, try and measure that benefit where possible, e.g. ‘Prediction accuracy was boosted by 15%.’
- Code and Notebooks: From your portfolio add links to codes hosted on services like GitHub or GitLab. It should be neat, uncluttered, documented and possess good readability.
- Visualizations: To make your results more effective and easily understandable, try to include such elements as interactive visualizations (dashboards created using Tableau or Plotly).
3. Data Analysis
Required skills involve analysis of data and report writing therefore candidates who express themselves well are preferred. It would help if you could apply all of these skills in any portfolio that you will create.
- Data Cleaning and Preparation: Demonstrate how you handle dirty data by explaining how you deal with missing values, how you transform data and how you engineer features in the datasets.
- Exploratory Data Analysis (EDA): Emphasize your ability to find patterns and identify patterns in graphical representations through libraries that include Matplotlib, Seaborn or ggplot2.
- Model Building and Evaluation: Incorporate every piece of work in which you have implemented and assessed the models of machine learning. For objective evaluations, it is recommended to provide precision accuracy or recall so that you can explain the performance of models.
- Data Storytelling: Make sure that you tell clear stories with your data and results and focus on what can and should be done. This is a foundation for closing the divide between technical tasks and decision-making.
4. Certifications and Achievements and Visualization Skills
Certifications and accolades support your expertise, and credibility assertion and prove the concept of lifelong learning.
- Professional Certifications: Some of the certifications to include are: Global Data Science Certified Professional, AWS Certified Data Analyst, and Google Data Analytics professional certificate.
- Academic Achievements: Post only courses, accomplishments or academic pursuits that give evidence of your academic learning.
- Hackathon Participation: Do not shy away from explaining your participation in sites like Kaggle or any other hackathons; stress on team spirit and as well as on innovative problem-solving abilities.
5. Personal Website or Blog
It may also be good to have a one-stop personal website that acts as a portfolio.
- Create a Professional Website: Select and build a website on WordPress, Wix, or, if it is possible, on GitHub pages to make its layout attractive and clear. Of particular information to attach are the introduction, the project information, the resume, and the contact information.
- Write Blog Posts: Promote learning by sharing what has been done or experienced, for example, difficulties encountered in projects or tasks, or tutorials about certain tools. This makes the firm a thought leader and also helps it in branding its image on the internet.
- Optimize for SEO: Use keywords such as data science portfolio templates or entry-level data analyst portfolio examples to make your portfolio easily accessible to employers.
Why TechAxis's Python with Data Science Course will help you?
The Python with Data Science course offered by TechAxis is your starting point towards becoming a successful Data Scientist. Tailored with clear goals, this course guarantees that you grasp the needed proficiency in various fields ranging from the basics of the Python programming language to the use of the machine learning approach. This is designed to help you gain the understanding and skills you need to thrive in the very cutthroat business of data science. The most attractive component of this course is the use of projects throughout the course. You study data science concepts on practical problems and not on hypothetical problems, which is very helpful in the sense you know what you will be facing once you join the job market.
The tutors are professionals who have accumulated considerable experience in their chosen fields; you get more than just lectures and thus make the learning experience more meaningful. Also, the course offers career support; you’ll learn what positions best fit you in data science as well as learn how you can write quality resumes for the positions and how to prepare for interviews. There is a concentration on portfolio development that will see you perfect in all your projects and expertise under mentors' supervision.
Whether you are fresh and want to get into the data science field, or you are an experienced professional who wants to upskill, TechAxis’s course in Python with Data Science is the best place to be with theoretical knowledge along with trainers with industry experience to help you succeed.
Conclusion
Pursuing a portfolio in data science is one of the important needs to address while on the job because it provides a unique way of presenting one’s ability, experience, and work to employers. Particularly important are IDs including written and verbal communication plans, selected projects, shown data analysis and visualization skills, obtained certifications, and professional presence on the web to make a story of your success. But what is a portfolio but an opportunity to demonstrate not merely lessons accrued but real capabilities to tackle tasks successfully? With data science remaining still vibrant and growing to cut across almost every sector, the need for people with appropriate skills in data science is however much higher than before. As a result, a well-developed portfolio helps market you as a strong contender to get the best positions, and it is high time to start creating it. If you are willing to go a step further, the Python with Data Science course offered at TechAxis is what you need. From the state of art curriculum, fun and challenging projects, and professional guidance, to portfolio assistance, you will transform into a confident candidate with all the abilities requisite to flourish in this innovative industry.