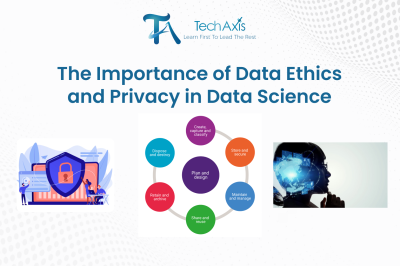
The Importance of Data Ethics and Privacy in Data Science
Just think for a moment about the prospect of having your data disclosed by an organization that you once trusted, and then having that data used to make certain decisions about you without having been consulted. More often than before, such ethical dilemmas arise in the present era of big data analytics. Since organizations are utilizing data science to deliver value across sectors, these practices need to be complementary to ethics, especially in privacy. Data science is the process by which large sets of data are collected, sorted through, and used to find patterns, decisions and answers. Its applications include everything from medical to electronic commerce.
Along with these prospects, there are also legal quirks such as algorithm bigotry, unlawful usage of data, and data breaches. Ethics in data science is applied to have appropriate standards for fairness, accountability as well as individual rights. Privacy being one of the cores holds personal information safeguarding, fostering trust and security. They are important to protect from harm, keep public trust, and encourage innovation in the long term. In this discussion, the contribution of ethical dilemmas to data science is discussed, examples of unethical using data are provided and data privacy is discussed as a society protection measure. Ethical practices can be both the best thing to do and the smart thing to do today and in the future handling the data.
Understanding the Ethical Implications of Data Science
Understanding the ethical implications of data science is crucial in today's data-driven world. Data science involves collecting, analyzing, and interpreting vast amounts of data, which can lead to significant societal impacts. Ethical concerns arise around issues like privacy, data security, algorithmic bias, and transparency. Misuse of data or flawed algorithms can reinforce discrimination, invade privacy, or exploit vulnerable populations. Therefore, data scientists must adopt responsible practices, ensuring fairness, accountability, and respect for user rights. By prioritizing ethical considerations, data science can drive progress while safeguarding individuals and society from potential harm.
Ethical Dilemmas in Data Science
Ethical dilemmas in data science arise when decisions involving data usage conflict with moral principles, such as privacy, fairness, and transparency. Issues like biased algorithms, unauthorized data collection, or misuse of sensitive information can lead to discrimination, privacy violations, and loss of trust. Addressing these dilemmas requires balancing innovation with ethical responsibility to ensure data-driven solutions are fair, accountable, and socially beneficial.
- Bias and Fairness: Any time, algorithms mirror the bias incorporated in the data offered throughout the training process. As an example, if the sample data is pre-biased against particular individuals, then the generated models may also be prejudiced, for example, hiring systems that inflame minorities. To maintain fairness all biases are to be looked for and removed during data pre-processing and modeling or selection of algorithms.
- Privacy Concerns: Personal information is often collected and processed in data science projects. When this data is collected without informed consent or when the collected data is used in a wrong way unrelated to the intended purpose it can be invasive of privacy and hence lack trust. Some examples of violation of the principles include monitoring the web activity without consent or transmitting personal health information.
- Transparency and Accountability: The complexity of some of the developments is such that many algorithms are opaque and determine outcomes autonomously. It means that such opacity can hide mistakes, perpetuate problematic functionality, and eventually undermine people’s trust. Thus, data scientists and organizations need to be very clear in documenting and being more accountable for their models.
- Security Risks: As data becomes an essential element in organizations, leakage and loss attract much attention. Any data that is not encrypted and kept safe can be used for wrong purposes if obtained including fiscal or medical records which can ruin a company’s reputation and leave it open to the law.
The Role of Data Scientists in Ethical Practices
The role of Data scientists is crucial in ensuring ethical practices by developing transparent, fair, and responsible data-driven solutions. They must identify and mitigate biases in algorithms, protect user privacy, and uphold data security while maintaining accountability in their work. By prioritizing ethical considerations, data scientists help build trust and ensure that their innovations benefit society without causing harm.
- Responsible Data Collection and Usage: It also means that data scientists need to make sure that the data is aggregated transparently, and that consent is obtained. They should refrain from employing data in a manner that violates either privacy or ethical standards – user rights should be respected.
- Ethical Model Development and Deployment: Ethical models should be made free of bias and should also be legal and ethical in their context. Deployment processes should place a premium on the welfare of the users and other people affected by the results of the modelling.
- Fair and Unbiased Algorithms: Data scientists, thus, need to think and act in a way that would prevent bias creep into algorithms. It also involves assessing training data for bias, checking the outputs for bias, and building models with a focus on devising fair and unbiased models.
Key Ethical Principles in Data Science
As we have discussed, good ethical attributes are core to the achievement of good practice of data science in that they set good practices from societal and legal perspectives. These are privacy by design, prohibition of discrimination, and openness and user control of content.
Privacy by Design
- Data Minimization: Limit data collection since restricting the use of data also reduces misuse of the data collected. This principle protects the privacy of the user and also minimizes the number of complications involved with handling data.
- Purpose Limitation: The anonymised data should not be used for another purpose for which the data had been gathered. Do not engage in secondary use unless permitted, which keeps relationships more trusting.
- Data Security: Unfortunately, by some failures, many enterprises become victims of hackers and cybercriminals, therefore sensitive data must be protected by encryption and secure access control to avoid compromise.
Equity and Non-Discrimination
- Avoiding Algorithmic Bias: Eliminate prejudices and discriminations from training data and models making sure they do not further the gap between the powerful and the rest. Just ideals are achieved by fair models for different groups of users.
- Ensuring Equal Treatment: Develop concepts and decision-making programs which are fair for everyone, and do not include discrimination by race, gender or socio-economic status.
Transparency And Accountability
- Explainable AI: When starting to design a good decision-making system, it is always wise to ensure that the systems being developed come with the added advantage of offering simple and easy-to-understand explanations of the decisions being made. Explicitly creates user trust and enables the assessment of fairness by the system.
- Auditing and Monitoring: Periodically review data management and results of algorithms to assess and prevent ethical lapses and address new problems early enough. Monitoring ensures how accountability is taken and how change is managed concerning new challenges.
In this way, the work of data scientists meets user rights, is fair and corresponds to the expectations of society according to the four principles outlined above. Business ethics go beyond being ethical in business, risk mitigation measures are also significantly important for gaining the public’s confidence and sustainability in data-driven businesses.
Real-World Examples of Data Ethics and Privacy Violations
- Case Study 1: Major Data Breach and Its Implication: Some remarkable data breaches happened in 2020 like a food delivery application in Nepal known as Foodmandu had their database hacked. This compromised other user information such as names, contacts, and delivery addresses among others. The situation revealed how weak Nepali organisations’ data protection measures are and urged them to provide proper cybersecurity that keeps the clients’ information safe and secure.
- Case Study 2: Discrimination in Algorithms Which Causes Discrimination: Some Nepali job portals just apply algorithms to screen the candidates with regard to their profiles. Despite this, there is evidence that they have biases, for example discriminating against candidates from rural areas even if they have been trained by candidates from urban areas. This raises an ethical question of how to fairly apply such algorithms to decide groups of people in a given socio-economic setting such as a Nepalese society.
- Case Study 3: Inadequate Policies in Data Collection and Processing: Some of the Nepali apps and websites running in recent years have been accused of harvesting users’ data beyond measure. For instance, A recent study produced by Stanford University researchers showed that some of the mob apps have been requesting for permissions which have no link with their actual functions but are rather linked to other issues that may cause misuse of user’s information. This has implications that require lucid privacy laws and corporate and individual app developer ethical policies.
Business Owners need to be aware of these data breach issues and thus must implement appropriate practices including the protection of the data, and fairness of algorithms to increase consumer trust and protect their rights in this increasingly technological society.
How to Promote Ethical Data Science Practices
Promoting ethical data science practices involves implementing clear guidelines and fostering a culture of responsibility. Organizations can establish ethical frameworks that prioritize fairness, transparency, and accountability in data collection, analysis, and usage. Providing training on bias detection, data privacy, and security helps data scientists identify and mitigate potential ethical risks. Additionally, encouraging collaboration among multidisciplinary teams, including ethicists and policymakers, ensures diverse perspectives in decision-making. By adopting these measures, organizations can ensure data science solutions are both innovative and socially responsible.
Ethical Guidelines and Codes of Conduct
- Follow the set IEEE or ACM code of ethical standards and ethical use of data in the industry.
- Set standards and continually monitor coping codes created internally for the organization and make sure the entire members of the organization follow ethical procedures in handling data.
Data Privacy Regulations
- Adhere to worldwide and neighbourhood information security provisions, for example, GDPR, and CCPA, to highlight duty regarding the accumulation and utilization of information ought to be taken under the Nepal Electronic Transactions Act.
- The performance should be checked, in particular, regarding the emerging changes to legal provisions.
Continuous Learning and Professional Development
- Help data scientists to attend workshops, training, and certification in Ethical data science.
- It is also important to monitor new threats and important practices in ethical usage of data, by active research and online groups.
- It is necessary to obey the law while training ethical practices in data science, which is important in order to avoid stimulating people’s distrust and undesired outcomes. Ethics needs to be an inherent aspect of data science programs in organizations to enable the formation of sustainable and valuable systems and business models for Society.
How TechAxis's Python with Data Science Course will help you?
TechAxis’s Python with Data Science program is designed for students aiming to become data scientists quickly and ethically. This course stands out due to its comprehensive curriculum, starting from the basics of Python programming to advanced machine learning, covering essential modules like data wrangling, visualization, and modelling. It emphasizes hands-on, real-world projects that focus on solving practical problems while addressing key issues such as bias and data protection. Led by expert instructors who are professional data scientists adhering to ethical standards, the course equips students to navigate ethical challenges in data science workflows.
Additionally, TechAxis provides career guidance services, including resume writing, interview preparation, and networking opportunities to help students discuss and implement ethical considerations in project development. A unique feature of the program is its focus on ethical hacking and data security, teaching students to safeguard valuable information against hackers and other malicious threats—an essential skill in today’s data-driven world. By combining technical training with social sensitivity, TechAxis’s Python with Data Science course prepares students to become successful and responsible data scientists. Join now to secure your future in this progressive and ethical field.
Conclusion
Responsible data science is founded on data ethics and privacy, which is the practice and process to avoid and mitigate items such as bias, privacy violation and secrecy. Overall, reacting ethically assists data professionals in avoiding bias, upholding equality, and protecting the data field from malicious actors; all while performing responsible social roles. Future concerns of data ethics will include increasing difficulty as the field continues to develop, with data ethicists being required always to learn and adapt.
It is for this reason, that for anyone who wants to succeed in this environment, knowledge and skills is key. TechAxis provides data science training that is as encyclopedic as it is ethical, and as thorough as it is privacy-conscious. This program ensures that through guidance from experts and practical experience, you are equipped with how you would handle ethical issues while implementing state-of-the-art and ethical data science practices.
Learn from TechAxis today and obtain a responsible data science professional geared towards transforming the future responsibly. Put ethics first in your projects and help to build a reliable and progressive data-oriented future.